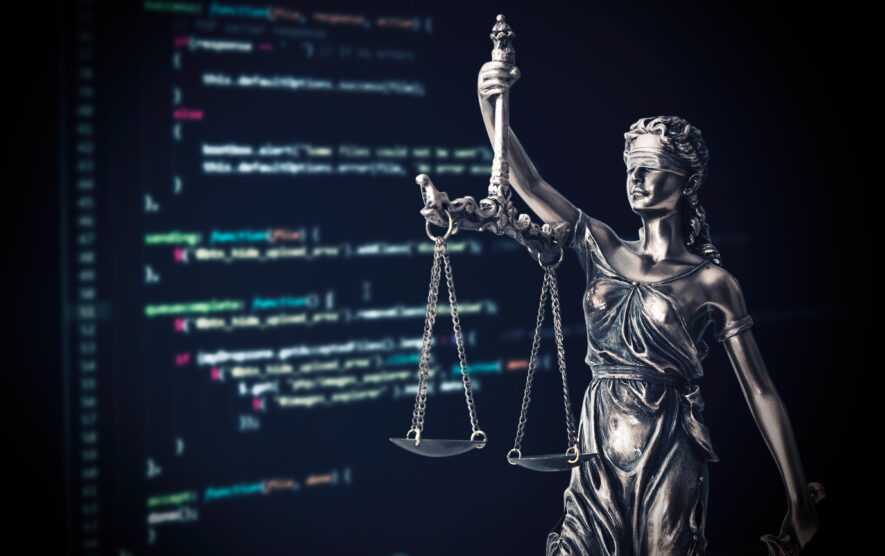
By Nils Klute, IT Editor and Project Manager Communication Cloud Services at EuroCloud Germany
To what extent neural networks can be protected under copyright law like databases, how machine learning algorithms differ from computer programmes in questions of intellectual property and at what point decentrally trained, artificially intelligent (AI) models can be a trade secret – a brief by HTWG Konstanz commissioned by the Karlsruhe Institute of Technology (KIT) explores legal questions surrounding federated learning.
Developing smart apps and implementing self-learning chatbots – a lack of data often slows down what is technologically possible, even in the Service-Meister project. One of the reasons for this is protection interests. For example, companies do not share their information with each other for fear of revealing trade secrets. Decentralised approaches like Federated Learning are supposed to solve the dilemma. Instead of data, companies exchange AI models.
Federated Learning: Know-how über KI modellieren und teilen
FLAIROP shows what this looks like in practice. The funding project was presented at the Digital Technology Days of the German Federal Minister for Economic Affairs in August 2022: In the project, so-called pick-and-place robots learn how to safely recognise and grasp known or unknown objects. Whether boxes, plastic bags or loosely packed individual parts – in order for the mechanical helpers to succeed faster and better, the German-Canadian consortium shares trained AI models via federated learning instead of information. “The robots exchange their know-how across the board,” said Jan R. Seyler of FLAIROP: “And that’s to learn not only from their own experience but from the knowledge of other robots.”
Trained AI models as trade secrets: KIT brief clarifies legal issues
Whether digital production facilities, connected cars or smart pick-and-place robots – if companies allow AI models to circulate across manufacturers, this makes self-learning and self-optimising applications possible. Applications that can develop independently via intelligent algorithms. And which thus simultaneously protect the interests with regard to their own data. What pleases companies, on the other hand, worries lawyers. So far, it is unclear what needs to be considered from a legal point of view when decentralised systems automatically generate knowledge and ultimately allow it to flow across company boundaries. Although the distributed federated learning approach does not exchange information, it does exchange know-how in the form of trained AI models. Such learned models can also be considered trade secrets. A current legal brief from Karlsruhe Institute of Technology (KIT) examines the issue. Lucas Zoller, Manuel Treiterer and Prof. Marc Strittmatter from the University of Applied Sciences Konstanz address legal protection aspects that become relevant for decentrally trained neural networks using the example of machine learning models. A fundamental problem: “Because data is not considered a thing due to its lack of embodiment, neither property rights nor rights of possession in the data as such can be established.”
Copyright aspects of Federated Learning
One possible solution is provided by copyright law, for example to protect databases as the result of an investment. The experts hold: “With the exception of the programmed network architecture, the legal examination comes to the conclusion that the considered components of neural networks are generally not likely to fulfil the requirements of the copyright protection rights in question. The special features of a federated learning process, in particular, have no effect on the existence of copyright protection in this respect.”
Trade secret holder: Multi-person constellations complicate determination
However, protection as a trade secret can be considered – and in principle for all components of a neural network. Nevertheless, questions remain open here as well, which arise through distributed approaches with multiple actors: “Due to the existing multi-person constellation within a Federated Learning process, difficulties also arise with regard to the classification as a trade secret holder.” Possible solutions include contractual agreements or neutral third parties as data trustees.
How should the protectability of untrained and trained AI models be legally assessed? What answers does the existing legal regime have in store for Federated Learning? The brief on the legal protectability of machine learning models using the example of decentrally trained neural networks is available for download (available in German only). KIT is an associated partner of Service-Meister.
Image credits: i-Stock-689948580
You liked this article? Then subscribe to our newsletter and receive regular updates on similar topics and the Project Service-Meister and discuss with us about this and similar exciting topics in our LinkedIn Group.