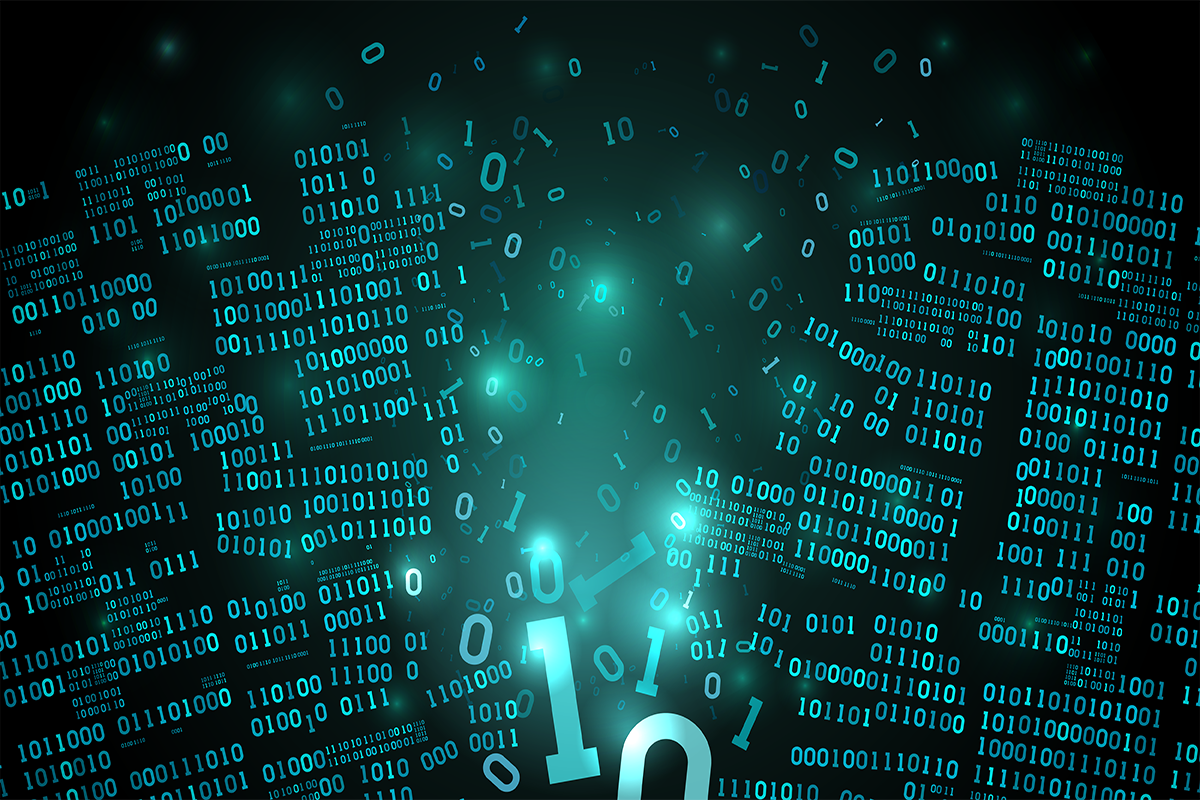
Author: Dr. Laura Ohrndorf, Data Scientist, grandcentrix GmbH
Optimising products, advising customers better and handling service cases faster – association analyses of service data make it possible. Which requirements have to be fulfilled, and which further advantages are offered to companies – grandcentrix and Würth report on the results of their work for Service-Meister in the blog post.
Whether it’s bookings, sales or returns – companies collect transaction data on an ongoing basis. This is data that is generated in business operations and maps financial, logistical and other business-related processes. Unlike other collected data, transaction data has a time stamp. If only for formal and legal reasons alone, operational or logistical data must be stored and archived. Often, this data is also used as a basis for analyses: descriptive analyses and the inclusion of insights derived from them in planning are more or less standard in most companies. Transaction data develops a particular potential when it is linked with other data records and filtered according to predefined criteria. This creates a new data set through intelligent processing. Subsequently, specific questions can be answered with various analysis methods. This approach is often called data discovery.
More than reporting and monitoring
Data discovery is about finding patterns and anomalies in consolidated data through analysis and visualisation. In contrast to classic BI data analysis, which focuses on reporting and monitoring the actual state of the data and forecasting based on this, data discovery goes further and tries to optimise business processes in a targeted manner with the help of different data sets or by breaking down data silos, identifying problems and answering questions.
Data discovery consists of three steps:
- Data Preparation: The preparation of data through cleansing (e.g. removing duplicates) and transformation.
- Data Visualisation: The visualisation of data using charts. – Advanced Analytics: The application of advanced analytical methods such as clustering or cohort analysis
Data Discovery in speedboat projects grandcentrix and Würth
The speedboat projects of grandcentrix and Würth follow the approach of Data Discovery with data sets on sales data and service cases. These are data on repair and maintenance processes that are handled in the in-house service workshop. With its extensive product and accessories portfolio, Würth offers a very valuable database that can be used to answer numerous questions about usage patterns and anomalies. Here, it is not only interesting how often individual products are purchased but also how the purchase is related to other purchases or even the use of services. With the help of association analysis, dependencies can be determined. In this way, important insights can be gained for optimising products, but also for advising customers and handling service cases.
Association analysis ensures customised offers
Association analysis is generally used to find patterns in data sets and to generate rules from them. The shopping basket analysis is particularly well known. Using existing transaction data, the habits of customers are analysed by calculating correlations between different goods and deriving associations from them. In most cases, suggestions for action are developed from the results. In this way, special offers can be made more effective or the arrangement of goods in the shop can be changed. In online shops, customers receive purchase suggestions based on association rules (“Customers who bought this product also bought …”).
While shopping basket analysis is the best-known application, association analysis is now used in numerous domains outside of marketing, for example, in the sequencing of viruses or the diagnosis of diseases.
Identifying associations between consumables and service cases
The speedboat projects focus on use cases involving service data. An analysis of which correlations exist between service cases is therefore obvious. In addition to detailed data on service cases and associated metadata, information on sales of equipment and orders for consumables such as screws, cut-off wheels or equipment accessories is available. For some devices, individual usage data or sensor values are also known so that these can also be included in an analysis.
All this data can be linked via the customer and serial number. Under the premise that customers buy equipment and accessories exclusively from Würth, a complete picture emerges of which equipment the customers use, which accessories they buy and, if they have equipment repaired or serviced, which equipment it was.
A first look at the visualised data, i.e. the second step of data discovery, can already yield interesting results here and provides initial indications of which data should be looked at in a more in-depth analysis.
Creation of association rules is the basis
Through targeted filtering and aggregation, very specific data sets can now be created that enable the generation of association rules. For example, a data set can be created in which the purchased consumables and possible service cases are recorded for owners of a certain tool. In this specific case, associations that have a repair as a consequence are particularly interesting. For example, the rule could be that customers who own a certain tool and buy a certain consumable material such as screws, drills or cutting discs are more likely to have a service case for this tool. The strength of this association can be defined using key figures.
Interpretation of the association rules by experts
Once the association rules have been established, the next step is to examine them for causality. Experts who are familiar with products and processes can help with this. Numerous scenarios are conceivable that result from a deeper analysis of the causes of defects, for example:
- Do defects occur particularly frequently with customers who bought a certain accessory?
- Is there a connection between the area of use and the frequency of defects?
- Do certain usage patterns of industries lead to certain types of defects?
All these questions lead to numerous actions that can not only prevent defects in the future but also increase customer satisfaction. The most obvious is the revision of equipment and accessories to adapt more closely to the real use of the equipment and to prevent failures through further development. It may be sufficient to revise instructions or recommendations. Often, a note on possibly incompatible accessories can also be integrated into the online shop.
Image credits: iStock-969859872
You liked this article? Then subscribe to our newsletter and receive regular updates on similar topics and the Project Service-Meister and discuss with us about this and similar exciting topics in our LinkedIn Group.